After defining what we mean by
data, it is helpful to consider what types of data you create and/or work with, and what format those data take. Your data stewardship practices will be dictated by the types of data that you work with, and what format they are in.
Data Types
Data types generally fall into five categories:
Observational
- Captured in situ
- Can’t be recaptured, recreated or replaced
- Examples: Sensor readings, sensory (human) observations, survey results
Experimental
- Data collected under controlled conditions, in situ or laboratory-based
- Should be reproducible, but can be expensive
- Examples: gene sequences, chromatograms, spectroscopy, microscopy
Derived or compiled
- Reproducible, but can be very expensive
- Examples: text and data mining, derived variables, compiled database, 3D models
Simulation
- Results from using a model to study the behavior and performance of an actual or theoretical system
- Models and metadata, where the input can be more important than output data
- Examples: climate models, economic models, biogeochemical models
Reference or canonical
- Static or organic collection [peer-reviewed] datasets, most probably published and/or curated.
- Examples: gene sequence databanks, chemical structures, census data, spatial data portals.
Data Formats
Research data comes in many varied formats: text, numeric, multimedia, models, software languages, discipline specific (e.g. crystallographic information file (CIF) in chemistry), and instrument specific.
Formats more likely to be accessible in the future are:
- Non-proprietary
- Open, documented standards
- In common usage by the research community
- Using standard character encodings (ASCII, UTF-8)
- Uncompressed (desirable, space permitting)
Use the table below to find an appropriate and recommended format for preserving and sharing your data over the long term.
TYPE OF DATA
|
PREFERRED FILE FORMATS FOR SHARING, RE-USE AND PRESERVATION
| Other Acceptable formats |
Quantitative tabular data with extensive metadata
- a dataset with variable labels, code labels, and defined missing values, in addition to the matrix of data
|
- SPSS portable format (.por)
- delimited text and command (‘setup’) file
- (SPSS, Stata, SAS, etc.) containing metadata information
- structured text or mark-up file containing metadata information, e.g. DDI XML file
|
MS Access (.mdb/.accdb)
|
Quantitative tabular data with minimal metadata
- a matrix of data with or without column headings or variable names, but no other metadata or labelling
|
- comma-separated values (CSV) file (.csv)
- tab-delimited file (.tab)
- including delimited text of given character set with SQL data definition statements where appropriate
|
- delimited text of given character set -- only characters not present in the data should be used as delimiters (.txt)
- widely-used formats, e.g. MS Excel (.xls/.xlsx), MS Access (.mdb/.accdb), dBase (.dbf) and OpenDocument Spreadsheet (.ods)
|
Geospatial data
vector and raster data
|
- ESRI Shapefile
- (essential: .shp, .shx, .dbf ; optional: .prj, .sbx, .sbn)
- geo-referenced TIFF (.tif, .tfw)
- CAD data (.dwg)
- tabular GIS attribute data
|
- ESRI Geodatabase format (.mdb)
- MapInfo Interchange Format (.mif) for vector data
|
Qualitative data
textual
|
- eXtensible Mark-up Language (XML) text according to an appropriate Document Type Definition (DTD) or schema (.xml)
- Rich Text Format (.rtf)
- plain text data, UTF-8 (Unicode; .txt)
|
- plain text data, ASCII (.txt)
- Hypertext Mark-up Language (HTML) (.html)
- widely-used proprietary formats, e.g. MS Word (.doc/.docx)
- LaTeX (.tex)
|
Digital image data
|
TIFF version 6 uncompressed (.tif)
|
- JPEG (.jpeg, .jpg)
- TIFF (other versions; .tif, .tiff)
- JPEG 2000 (.jp2)
- Adobe Portable Document Format (PDF/A,
PDF) (.pdf)
|
Digital audio data
|
- Free Lossless Audio Codec (FLAC) (.flac)
- Waveform Audio Format (WAV) (.wav)
- MPEG-1 Audio Layer 3 (.mp3) - spoken word audio only
|
- MPEG-1 Audio Layer 3 (.mp3)
- Audio Interchange File Format (AIFF) (.aif)
|
Digital video data
|
- MPEG-4 High Profile (.mp4)
- motion JPEG 2000 (.jp2)
|
JPEG 2000 (.mj2)
|
Documentation & Scripts
|
- Rich Text Format (.rtf)
- Open Document Text (.odt)
- HTML (.htm, .html)
|
- plain text (.txt)
- widely-used proprietary formats, e.g. MS Word (.doc/.docx) or MS Excel (.xls/ .xlsx)
- XML marked-up text (.xml) according to an appropriate DTD or schema, e.g. XHMTL 1.0
- PDF/A or PDF (.pdf)
|
Chemistry data
spectroscopy data and other plots which require the capability of representing contours as well as peak position and intensity
|
Convert NMR, IR, Raman, UV and Mass Spectrometry files to JCAMP format for ease in sharing.
|
Introduction
If you have been part of the
data science (or any data!) industry, you would know the challenge of working with different data types. Different formats, different compression, different parsing on different systems – you could be quickly pulling your hair! Oh and I have not talked about the unstructured data or semi-structured data yet.
For any data scientist or data engineer, dealing with different formats can become a tedious task. In real-world, people rarely get neat tabular data. Thus, it is mandatory for any data scientist (or a data engineer) to be aware of different file formats, common challenges in handling them and the best / efficient ways to handle this data in real life.
This article provides common formats a data scientist or a data engineer must be aware of. I will first introduce you to different common file formats used in the industry. Later, we’ll see how to read these file formats in
Python.
P.S. In rest of this article, I will be referring to a data scientist, but the same applies to a data engineer or any data science professional.
Table of Contents
- What is a file format?
- Why should a data scientist understand different file formats?
- Different file formats and how to read them in Python?
- Comma-separated values
- XLSX
- ZIP
- Plain Text (txt)
- JSON
- XML
- HTML
- Images
- Hierarchical Data Format
- PDF
- DOCX
- MP3
- MP4
1. What is a file format?
A file format is a standard way in which information is encoded for storage in a file. First, the file format specifies whether the file is a binary or ASCII file. Second, it shows how the information is organized. For example, comma-separated values (CSV) file format stores tabular data in plain text.
To identify a file format, you can usually look at the file extension to get an idea. For example, a file saved with name “Data” in “CSV” format will appear as “Data.csv”. By noticing “.csv” extension we can clearly identify that it is a “CSV” file and data is stored in a tabular format.
2. Why should a data scientist understand different file formats?
Usually, the files you will come across will depend on the application you are building. For example, in an image processing system, you need image files as input and output. So you will mostly see files in jpeg, gif or png format.
As a data scientist, you need to understand the underlying structure of various file formats, their advantages and dis-advantages. Unless you understand the underlying structure of the data, you will not be able to explore it. Also, at times you need to make decisions about how to store data.
Choosing the optimal file format for storing data can improve the performance of your models in data processing.
Now, we will look at the following file formats and how to read them in Python:
- Comma-separated values
- XLSX
- ZIP
- Plain Text (txt)
- JSON
- XML
- HTML
- Images
- Hierarchical Data Format
- PDF
- DOCX
- MP3
- MP4
3. Different file formats and how to read them in Python
3.1 Comma-separated values
Comma-separated values file format falls under spreadsheet file format.
What is Spreadsheet File Format?
In spreadsheet file format, data is stored in cells. Each cell is organized in rows and columns. A column in the spreadsheet file can have different types. For example, a column can be of string type, a date type or an integer type. Some of the most popular spreadsheet file formats are Comma Separated Values ( CSV ), Microsoft Excel Spreadsheet ( xls ) and Microsoft Excel Open XML Spreadsheet ( xlsx ).
Each line in CSV file represents an observation or commonly called a record. Each record may contain one or more fields which are separated by a comma.
Sometimes you may come across files where fields are not separated by using a comma but they are separated using tab. This file format is known as TSV (Tab Separated Values) file format.
The below image shows a CSV file which is opened in Notepad.
Reading the data from CSV in Python
Let us look at how to read a CSV file in Python. For loading the data you can use the “pandas” library in python.
import pandas as pd
df = pd.read_csv(“/home/Loan_Prediction/train.csv”)
Above code will load the train.csv file in DataFrame df.
3.2 XLSX files
XLSX is a Microsoft Excel Open XML file format. It also comes under the Spreadsheet file format. It is an XML-based file format created by Microsoft Excel. The XLSX format was introduced with Microsoft Office 2007.
In XLSX data is organized under the cells and columns in a sheet. Each XLSX file may contain one or more sheets. So a workbook can contain multiple sheets.
The below image shows a “xlsx” file which is opened in Microsoft Excel.
In above image, you can see that there are multiple sheets present (bottom left) in this file, which are Customers, Employees, Invoice, Order. The image shows the data of only one sheet – “Invoice”.
Reading the data from XLSX file
Let’s load the data from XLSX file and define the sheet name. For loading the data you can use the Pandas library in python.
import pandas as pd
df = pd.read_excel(“/home/Loan_Prediction/train.xlsx”, sheetname = “Invoice”)
Above code will load the sheet “Invoice” from “train.xlsx” file in DataFrame df.
3.3 ZIP files
ZIP format is an archive file format.
What is Archive File format?
In Archive file format, you create a file that contains multiple files along with metadata. An archive file format is used to collect multiple data files together into a single file. This is done for simply compressing the files to use less storage space.
There are many popular computer data archive format for creating archive files. Zip, RAR and Tar being the most popular archive file format for compressing the data.
So, a ZIP file format is a lossless compression format, which means that if you compress the multiple files using ZIP format you can fully recover the data after decompressing the ZIP file. ZIP file format uses many compression algorithms for compressing the documents. You can easily identify a ZIP file by the .zip extension.
Reading a .ZIP file in Python
You can read a zip file by importing the “zipfile” package. Below is the python code which can read the “train.csv” file that is inside the “T.zip”.
import zipfile
archive = zipfile.ZipFile('T.zip', 'r')
df = archive.read('train.csv')
Here, I have discussed one of the famous archive format and how to open it in python. I am not mentioning other archive formats. If you want to read about different archive formats and their comparisons you can refer this
link.
3.4 Plain Text (txt) file format
In Plain Text file format, everything is written in plain text. Usually, this text is in unstructured form and there is no meta-data associated with it. The txt file format can easily be read by any program. But interpreting this is very difficult by a computer program.
Let’s take a simple example of a text File.
The following example shows text file data that contain text:
“In my previous article, I introduced you to the basics of Apache Spark, different data representations
(RDD / DataFrame / Dataset) and basics of operations (Transformation and Action). We even solved a machine
learning problem from one of our past hackathons. In this article, I will continue from the place I left in
my previous article. I will focus on manipulating RDD in PySpark by applying operations
(Transformation and Actions).”
Suppose the above text written in a file called text.txt and you want to read this so you can refer the below code.
text_file = open("text.txt", "r")
lines = text_file.read()
3.5 JSON file format
JavaScript Object Notation(JSON) is a text-based open standard designed for exchanging the data over web. JSON format is used for transmitting structured data over the web. The JSON file format can be easily read in any programming language because it is language-independent data format.
Let’s take an example of a JSON file
The following example shows how a typical JSON file stores information of employees.
{
"Employee": [
{
"id":"1",
"Name": "Ankit",
"Sal": "1000",
},
{
"id":"2",
"Name": "Faizy",
"Sal": "2000",
}
]
}
Reading a JSON file
Let’s load the data from JSON file. For loading the data you can use the pandas library in python.
import pandas as pd
df = pd.read_json(“/home/kunal/Downloads/Loan_Prediction/train.json”)
3.6 XML file format
XML is also known as Extensible Markup Language. As the name suggests, it is a markup language. It has certain rules for encoding data. XML file format is a human-readable and machine-readable file format. XML is a self-descriptive language designed for sending information over the internet. XML is very similar to HTML, but has some differences. For example, XML does not use predefined tags as HTML.
Let’s take the simple example of XML File format.
The following example shows an xml document that contains the information of an employee.
<?xml version="1.0"?>
<contact-info>
<name>Ankit</name>
<company>Anlytics Vidhya</company>
<phone>+9187654321</phone>
</contact-info>
The “<?xml version=”1.0″?>” is a XML declaration at the start of the file (it is optional). In this deceleration, version specifies the XML version and encoding specifies the character encoding used in the document. <contact-info> is a tag in this document. Each XML-tag needs to be closed.
Reading XML in python
For reading the data from XML file you can import xml.etree. ElementTree library.
Let’s import an xml file called train and print its root tag.
import xml.etree.ElementTree as ET
tree = ET.parse('/home/sunilray/Desktop/2 sigma/train.xml')
root = tree.getroot()
print root.tag
3.7 HTML files
HTML stands for Hyper Text Markup Language. It is the standard markup language which is used for creating Web pages. HTML is used to describe structure of web pages using markup. HTML tags are same as XML but these are predefined. You can easily identify HTML document subsection on basis of tags such as <head> represent the heading of HTML document. <p> “paragraph” paragraph in HTML. HTML is not case sensitive.
The following example shows an HTML document.
<!DOCTYPE html>
<html>
<head>
<title>Page Title</title>
</head>
<body><h1>My First Heading</h1>
<p>My first paragraph.</p></body>
</html>
Each tag in HTML is enclosed under the angular bracket(<>). The <!DOCTYPE html> tag defines that document is in HTML format. <html> is the root tag of this document. The <head> element contains heading part of this document. The <title>, <body>, <h1>, <p> represent the title, body, heading and paragraph respectively in the HTML document.
Reading the HTML file
3.8 Image files
Image files are probably the most fascinating file format used in data science. Any computer vision application is based on image processing. So it is necessary to know different image file formats.
Usual image files are 3-Dimensional, having RGB values. But, they can also be 2-Dimensional (grayscale) or 4-Dimensional (having intensity) – an Image consisting of pixels and meta-data associated with it.
Each image consists one or more frames of pixels. And each frame is made up of two-dimensional array of pixel values. Pixel values can be of any intensity. Meta-data associated with an image, can be an image type (.png) or pixel dimensions.
Let’s take the example of an image by loading it.
from scipy import misc
f = misc.face()
misc.imsave('face.png', f) # uses the Image module (PIL)
import matplotlib.pyplot as plt
plt.imshow(f)
plt.show()
Now, let’s check the type of this image and its shape.
type(f) , f.shape
numpy.ndarray,(768, 1024, 3)
3.9 Hierarchical Data Format (HDF)
In Hierarchical Data Format ( HDF ), you can store a large amount of data easily. It is not only used for storing high volumes or complex data but also used for storing small volumes or simple data.
The advantages of using HDF are as mentioned below:
- It can be used in every size and type of system
- It has flexible, efficient storage and fast I/O.
- Many formats support HDF.
There are multiple HDF formats present. But, HDF5 is the latest version which is designed to address some of the limitations of the older HDF file formats. HDF5 format has some similarity with XML. Like XML, HDF5 files are self-describing and allow users to specify complex data relationships and dependencies.
Let’s take the example of an HDF5 file format which can be identified using .h5 extension.
Read the HDF5 file
You can read the HDF file using pandas. Below is the python code can load the train.h5 data into the “t”.
t = pd.read_hdf(‘train.h5’)
3.10 PDF file format
PDF (Portable Document Format) is an incredibly useful format used for interpretation and display of text documents along with incorporated graphics. A special feature of a PDF file is that it can be secured by a password.
Here’s an example of a pdf file.
Reading a PDF file
On the other hand, reading a PDF format through a program is a complex task. Although there exists a library which do a good job in parsing PDF file, one of them is PDFMiner. To read a PDF file through PDFMiner, you have to:
- Download PDFMiner and install it through the website
- Extract PDF file by the following code
pdf2txt.py <pdf_file>.pdf
3.11 DOCX file format
Microsoft word docx file is another file format which is regularly used by organizations for text based data. It has many characteristics, like inline addition of tables, images, hyperlinks, etc. which helps in making docx an incredibly important file format.
The advantage of a docx file over a PDF file is that a docx file is editable. You can also change a docx file to any other format.
Here’s an example of a docx file:
Reading a docx file
Similar to PDF format, python has a community contributed library to parse a docx file. It is called python-docx2txt.
Installing this library is easy through pip by:
pip install docx2txt
To read a docx file in Python use the following code:
import docx2txt
text = docx2txt.process("file.docx")
3.12 MP3 file format
MP3 file format comes under the multimedia file formats. Multimedia file formats are similar to image file formats, but they happen to be one the most complex file formats.
In multimedia file formats, you can store variety of data such as text image, graphical, video and audio data. For example, A multimedia format can allow text to be stored as Rich Text Format (RTF) data rather than ASCII data which is a plain-text format.
MP3 is one of the most common audio coding formats for digital audio. A mp3 file format uses the MPEG-1 (Moving Picture Experts Group – 1) encoding format which is a standard for lossy compression of video and audio. In lossy compression, once you have compressed the original file, you cannot recover the original data.
A mp3 file format compresses the quality of audio by filtering out the audio which can not be heard by humans. MP3 compression commonly achieves 75 to 95% reduction in size, so it saves a lot of space.
mp3 File Format Structure
A mp3 file is made up of several frames. A frame can be further divided into a header and data block. We call these sequence of frames an elementary stream.
A header in mp3 usually, identify the beginning of a valid frame and a data blocks contain the (compressed) audio information in terms of frequencies and amplitudes. If you want to know more about mp3 file structure you can refer this
link.
Reading the multimedia files in python
For reading or manipulating the multimedia files in Python you can use a library called
PyMedia.
3.13 MP4 file format
MP4 file format is used to store videos and movies. It contains multiple images (called frames), which play in form of a video as per a specific time period. There are two methods for interpreting a mp4 file. One is a closed entity, in which the whole video is considered as a single entity. And other is mosaic of images, where each image in the video is considered as a different entity and these images are sampled from the video.
Here’s is an example of mp4 video
Reading an mp4 file
MP4 also has a community built library for reading and editing mp4 files, called MoviePy.
You can install the library from this
link. To read a mp4 video clip, in Python use the following code.
from moviepy.editor import VideoFileClip
clip = VideoFileClip(‘<video_file>.mp4’)
You can then display this in jupyter notebook as below
ipython_display(clip)
End Notes
In this article, I have introduced you to some of the basic file formats, which are used by data scientist on a day to day basis. There are many file formats I have not covered. Good thing is that I don’t need to cover all of them in one article.
I hope you found this article helpful. I would encourage you to explore more file formats. Good luck! If you still have any difficulty in understanding a specific data format, I’d like to interact with you in comments. If you have any more doubts or queries feel free to drop in your comments below.
There is a subtle difference between data and information. Data are the facts or details from which information is derived. Individual pieces of data are rarely useful alone. For data to become information, data needs to be put into context.
Comparison chart
Data versus Information comparison chart
| Data | Information |
Meaning | Data is raw, unorganized facts that need to be processed. Data can be something simple and seemingly random and useless until it is organized. | When data is processed, organized, structured or presented in a given context so as to make it useful, it is called information. |
Example | Each student's test score is one piece of data. | The average score of a class or of the entire school is information that can be derived from the given data. |
Etymology | "Data" comes from a singular Latin word, datum, which originally meant "something given." Its early usage dates back to the 1600s. Over time "data" has become the plural of datum. | "Information" is an older word that dates back to the 1300s and has Old French and Middle English origins. It has always referred to "the act of informing, " usually in regard to education, instruction, or other knowledge communication. |
Data vs. Information - Differences in Meaning
Data are simply facts or figures — bits of information, but not information itself. When data are processed, interpreted, organized, structured or presented so as to make them meaningful or useful, they are called information. Information provides context for data.
For example, a list of dates — data — is meaningless without the information that makes the dates relevant (dates of holiday).
"Data" and "information" are intricately tied together, whether one is recognizing them as two separate words or using them interchangeably, as is common today. Whether they are used interchangeably depends somewhat on the usage of "data" — its context and
grammar.
Examples of Data and Information
- The history of temperature readings all over the world for the past 100 years is data. If this data is organized and analyzed to find that global temperature is rising, then that is information.
- The number of visitors to a website by country is an example of data. Finding out that traffic from the U.S. is increasing while that from Australia is decreasing is meaningful information.
- Often data is required to back up a claim or conclusion (information) derived or deduced from it. For example, before a drug is approved by the FDA, the manufacturer must conduct clinical trials and present a lot of data to demonstrate that the drug is safe.
"Misleading" Data
Because data needs to be interpreted and analyzed, it is quite possible — indeed, very probable — that it will be interpreted incorrectly. When this leads to erroneous conclusions, it is said that the data are misleading. Often this is the result of incomplete data or a lack of context. For example, your investment in a
mutual fund may be up by 5% and you may conclude that the fund managers are doing a great job. However, this could be misleading if the major stock market indices are up by 12%. In this case, the fund has underperformed the market significantly.
What is Data?
Data is a raw and unorganized fact that required to be processed to make it meaningful. Data can be simple at the same time unorganized unless it is organized. Generally, data comprises facts, observations, perceptions numbers, characters, symbols, image, etc.
Data is always interpreted, by a human or machine, to derive meaning. So, data is meaningless. Data contains numbers, statements, and characters in a raw form.
What is Information?
Information is a set of data which is processed in a meaningful way according to the given requirement. Information is processed, structured, or presented in a given context to make it meaningful and useful.
It is processed data which includes data that possess context, relevance, and purpose. It also involves manipulation of raw data.
Information assigns meaning and improves the reliability of the data. It helps to ensure undesirability and reduces uncertainty. So, when the data is transformed into information, it never has any useless details.
Data Vs. Information
Parameters | Data | Information |
Description | Qualitative Or QuantitativeVariables which helps to develop ideas or conclusions. | It is a group of data which carries news and meaning. |
Etymology | Data comes from a Latin word, datum, which means "To give something." Over a time "data" has become the plural of datum. | Information word has old French and middle English origins. It has referred to the "act of informing.". It is mostly used for education or other known communication. |
Format | Data is in the form of numbers, letters, or a set of characters. | Ideas and inferences |
Represented in | It can be structured, tabular data, graph, data tree, etc. | Language, ideas, andthoughts based on the given data. |
Meaning | Data does not have any specific purpose. | It carries meaning that has been assigned by interpreting data. |
Interrelation | Information that is collected | Information that is processed. |
Feature | Data is a single unit and is raw. It alone doesn't have any meaning. | Information is the product and group of data which jointly carry a logical meaning. |
Dependence | It never depends on Information | It depended on Data. |
Measuring unit | Measured in bits and bytes. | Measured in meaningful units like time, quantity, etc. |
Support for Decision making | It can't be used for decision making | It is widely used for decision making. |
Contains | Unprocessed raw factors | Processed in a meaningful way |
Knowledge level | It is low-level knowledge. | It is the second level of knowledge. |
Characteristic | Data is the property of an organization and is not available for sale to the public. | Information is available for sale to the public. |
Dependency | Data depends upon the sources for collecting data. | Information depends upon data. |
Example | Ticket sales on a band on tour. | Sales report by region and venue. It gives information which venue is profitable for that business. |
Significance | Data alone has no signifiance. | Information is significant by itself. |
Meaning | Data is based on records and observations and, which are stored in computers or remembered by a person. | Information is considered more reliable than data. It helps the researcher to conduct a proper analysis. |
Usefulness | The data collected by the researcher, may or may not be useful. | Information is useful and valuable as it is readily available to the researcher for use. |
Dependency | Data is never designed to the specific need of the user. | Information is always specific to the requirements and expectations because all the irrelevant facts and figures are removed, during the transformation process. |
DIKW (Data Information Knowledge Wisdom)
Conclusion
- Data is a raw and unorganized fact that required to be processed to make it meaningful.
- Information is a set of data which is processed in a meaningful way according to the given requirement.
- Data comes from a Latin word, datum, which means "To give something."
- Information word has old French and middle English origins. It has referred to the "act of informing.".
- Data is in the form of numbers, letters, or a set of characters.
- Information is mainly in the form of Ideas and inferences.
- DIKW is the model used for discussion of data, information, knowledge, wisdom and their interrelationships
Research process starts with the collection of data, which plays a significant role in the statistical analysis. We quite commonly use the term ‘data’ in the different context. However, in general, it indicates the facts or statistics gathered by the researcher for analysis in their original form. When the data is processed and transformed in such a way that it becomes useful to the users, it is known as ‘information’.
While data is an unsystematic fact or detail about something, information is a systematic and filtered form of data, which is useful. In this articl, you can find all the important differences between data and information.
Content: Data Vs Information
- Comparison Chart
- Definition
- Key Differences
- Conclusion
Comparison Chart
BASIS FOR COMPARISON | DATA | INFORMATION |
Meaning | Data means raw facts gathered about someone or something, which is bare and random. | Facts, concerning a particular event or subject, which are refined by processing is called information. |
What is it? | It is just text and numbers. | It is refined data. |
Based on | Records and Observations | Analysis |
Form | Unorganized | Organized |
Useful | May or may not be useful. | Always |
Specific | No | Yes |
Dependency | Does not depend on information. | Without data, information cannot be processed. |
Definition of Data
Data is defined as the collection of facts and details like text, figures, observations, symbols or simply description of things, event or entity gathered with a view to drawing inferences. It is the raw fact, which should be processed to gain information. It is the unprocessed data, that contains numbers, statements and characters before it is refined by the researcher
The term data is derived from Latin term ‘datum’ which refers to ‘something given’. The concept of data is connected with scientific research, which is collected by various organisations, government departments, institutions and non-government agencies for a variety of reasons. There can be two types of data:
Primary Data
Qualitative Data
Quantitative Data
Secondary Data
Internal Data
External Data
Definition of Information
Information is described as that form of data which is processed, organised, specific and structured, which is presented in the given setting. It assigns meaning and improves the reliability of the data, thus ensuring understandability and reduces uncertainty. When the data is transformed into information, it is free from unnecessary details or immaterial things, which has some value to the researcher.
The term information discovered from the Latin word ‘informare’, which refers to ‘give form to’. Raw data is not at all meaningful and useful as information. It is refined and cleaned through purposeful intelligence to become information. Therefore data is manipulated through tabulation, analysis and similar other operations which enhance the explanation and interpretation.
Key Differences Between Data and Information
The points given below are substantial, so far as the difference between data and information is concerned:
Raw facts gathered about a condition, event, idea, entity or anything else which is bare and random, is called data. Information refers to facts concerning a particular event or subject, which are refined by processing.
Data are simple text and numbers, while information is processed and interpreted data.
Data is in an unorganized form, i.e. it is randomly collected facts and figures which are processed to draw conclusions. On the other hand, when the data is organised, it becomes information, which presents data in a better way and gives meaning to it.
Data is based on observations and records, which are stored in computers or simply remembered by a person. As against this, information is considered more reliable than data, as a proper analysis is conducted to convert data into information by the researcher or investigator.
The data collected by the researcher, may or may not be useful to him, as when the data is gathered, it is not known what they are about or what they represent? Conversely, information is valuable and useful to the researcher because it is presented in the given context and so readily available to the researcher for use.
Data is not always specific to the need of the researcher, but information is always specific to his requirements and expectations, because all the irrelevant facts and figures are eliminated, during the transformation of data into information.
When it comes to dependency, data does not depend on information. However, information cannot exist without data.
Conclusion
In simple terms, data is unorganised information and information is processed data. These two terms are so closely intertwined that it is quite common for people to juxtapose them. In the technical glossary, data means input, used to generate output, i.e. information.
Data are those facts and descriptions from which information can be extracted. Data alone has no certain meaning, i.e. until and unless the data is explained and interpreted, it is just a collection of numbers, words and symbols. Unlike information, which does not lack meaning in fact they can be understood by the users in normal diligence.
Data collection plays a very crucial role in the statistical analysis. In research, there are different methods used to gather information, all of which fall into two categories, i.e. primary data, and secondary data. As the name suggests, primary data is one which is collected for the first time by the researcher while secondary data is the data already collected or produced by others
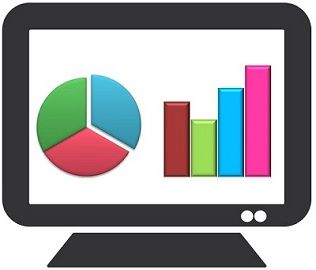
Data collection plays a very crucial role in the statistical analysis. In research, there are different methods used to gather information, all of which fall into two categories, i.e. primary data, and secondary data. As the name suggests, primary data is one which is collected for the first time by the researcher while secondary data is the data already collected or produced by others.
There are many differences between primary and secondary data, which are discussed in this article. But the most important difference is that primary data is factual and original whereas secondary data is just the analysis and interpretation of the primary data. While primary data is collected with an aim for getting solution to the problem at hand, secondary data is collected for other purposes.
Content: Primary Data Vs Secondary Data
- Comparison Chart
- Definition
- Key Differences
- Conclusion
Comparison Chart
BASIS FOR COMPARISON | PRIMARY DATA | SECONDARY DATA |
Meaning | Primary data refers to the first hand data gathered by the researcher himself. | Secondary data means data collected by someone else earlier. |
Data | Real time data | Past data |
Process | Very involved | Quick and easy |
Source | Surveys, observations, experiments, questionnaire, personal interview, etc. | Government publications, websites, books, journal articles, internal records etc. |
Cost effectiveness | Expensive | Economical |
Collection time | Long | Short |
Specific | Always specific to the researcher's needs. | May or may not be specific to the researcher's need. |
Available in | Crude form | Refined form |
Accuracy and Reliability | More | Relatively less |
Definition of Primary Data
Primary data is data originated for the first time by the researcher through direct efforts and experience, specifically for the purpose of addressing his research problem. Also known as the first hand or raw data. Primary data collection is quite expensive, as the research is conducted by the organisation or agency itself, which requires resources like investment and manpower. The data collection is under direct control and supervision of the investigator.
The data can be collected through various methods like surveys, observations, physical testing, mailed questionnaires, questionnaire filled and sent by enumerators, personal interviews, telephonic interviews, focus groups, case studies, etc.
Definition of Secondary Data
Secondary data implies second-hand information which is already collected and recorded by any person other than the user for a purpose, not relating to the current research problem. It is the readily available form of data collected from various sources like censuses, government publications, internal records of the organisation, reports, books, journal articles, websites and so on.
Secondary data offer several advantages as it is easily available, saves time and cost of the researcher. But there are some disadvantages associated with this, as the data is gathered for the purposes other than the problem in mind, so the usefulness of the data may be limited in a number of ways like relevance and accuracy.
Moreover, the objective and the method adopted for acquiring data may not be suitable to the current situation. Therefore, before using secondary data, these factors should be kept in mind.
Key Differences Between Primary and Secondary Data
The fundamental differences between primary and secondary data are discussed in the following points:
The term primary data refers to the data originated by the researcher for the first time. Secondary data is the already existing data, collected by the investigator agencies and organisations earlier.
Primary data is a real-time data whereas secondary data is one which relates to the past.
Primary data is collected for addressing the problem at hand while secondary data is collected for purposes other than the problem at hand.
Primary data collection is a very involved process. On the other hand, secondary data collection process is rapid and easy.
Primary data collection sources include surveys, observations, experiments, questionnaire, personal interview, etc. On the contrary, secondary data collection sources are government publications, websites, books, journal articles, internal records etc.
Primary data collection requires a large amount of resources like time, cost and manpower. Conversely, secondary data is relatively inexpensive and quickly available.
Primary data is always specific to the researcher’s needs, and he controls the quality of research. In contrast, secondary data is neither specific to the researcher’s need, nor he has control over the data quality.
Primary data is available in the raw form whereas secondary data is the refined form of primary data. It can also be said that secondary data is obtained when statistical methods are applied to the primary data.
Data collected through primary sources are more reliable and accurate as compared to the secondary sources.
Conclusion
As can be seen from the above discussion that primary data is an original and unique data, which is directly collected by the researcher from a source according to his requirements. As opposed to secondary data which is easily accessible but are not pure as they have undergone through many statistical treatments.
Data and Information are interrelated, as the data is the basic building block for the later. But, there are various key points that differ from each other.
Data is something that you can consider as the low level of knowledge. In this, you have some scattered, Uncategorized, unorganized entities that do not really mean anything. Whereas Information is the second level of knowledge where you wire up the data and assign them some context. So that, the data become meaningful.
Most of the people are aware of the data and information but still, there is some ambiguity in people about the difference between the data and information.
In this article, I am going to provide a brief explanation of what data and information are. Also, in this article, you’ll get to know the key difference between the two.
So, before differentiating the two on the basis of several factors, let me first throw some light on what data and information are.
What is data?
data
The term data was originated around the 1600s that comes from a singular Latin word datum, which means “something given”.
The dictionary meaning of the word data is, “Facts and statistics collected together for reference or analysis”.
And according to the philosophy data means, “Things known or assumed as facts, making the basis of reasoning or calculation”.
*Reference: According to the Oxford dictionary
And generally, we say, “Data is a collection of raw facts and figures that we need to process to extract meaning or information”.
As per the definition, data is something that we have as raw entities. These entities can be any number(0 to 9), characters (A to Z, a to z), text, words, statements or even special characters (*, /, @, #, etc…).
Furthermore, pictures, sound, or videos that are contextless, means little or nothing to a human being and lies in the category of data. Example: Will Turner, 48, link down, blue, junior, ocean, street
Data is nothing unless it is processed or it is aligned in some context. The data when structured and organized i.e; when the data is processed in some manner then the result or the output is the Information.
As for the above example, the data is raw and there is no meaning to it but if we organize this data:
Will tuner
H.no- 48, blue ocean
link down street
Now, this looks like an Adress of the person named Will Turner. Whereas, in the above example it is impossible to make out a meaning of the words.
What is information?
The term information was originated around the 1300s i.e; before the term data. The information word comes from a singular old English word informare, which refers to “the act of informing”.
The dictionary meaning of the word information is, “knowledge gained through study, communication, research, instruction, etc.”.
In term of computers, the term information means, “important or useful facts obtained as output from a computer by means of processing input data with a program”.
*Reference: According to the dictionary
Apart from these, generally we consider information as, “a processed data that is organized, structured or presented in a given context so that the data deliver some logical meaning that may be further utilized in decision making”.
The information that you get after the processing of data is abstract and free from any sort of unnecessary details. This information is precise and conveys a straightforward meaning to the output that you get from the processing of the raw and meaningless data.
The information you get from the processing of data is utilized for some further judgment.
The information has four main uses that are as following:
1. Planning
To plan accurately, a business must know the resources it has. Example: People, Properties, clients, customers, dealers, Types of machinery, Accounts etc.
With all the above information it becomes easier for the business to look into the market and plan strategies to overtake the actions of the competitors.
At the planning stage, the information is the key ingredient in business level decision making.
2. Recording
Recording of each transaction and event is must for a business. It is important to record the information like the expenses and income as per the law for management of the taxes. A business also keeps rethe cord of the marketing and the sale or purchase of the products so that they can keep track of customers behavior about the purchase of products.
If we say in other contexts like school and universities keep track of the number of admissions per year and the number of students that pass out per year to make further decisions.
3. Analysis
You can utilize this information for the analysis purpose. The analysis includes the sales, costs, and profits etc.
The analysis gives a broad figure of the overall profit or loss of the organization. Based on this analysis the business can make decisions that will optimize the costs and the profits in the best way.
4. Controlling
Once, a business has all the record data and the overall analysis, then it will be easier for it to control and enhance the resources.
Hence, the information helps in identifying whether the things are going better or worse than the expectation.
Accordingly, the business can control the expenses and manage resources to attain what is in the expectation.
The information that is in the use of decision making purpose are of three types:
Strategic information: This information helps in planning the objectives of an organization. It also helps to measure the achievements of the objectives.
Tactical Information: This information decides how to employ the resources to attain the maximum productivity.
Operational Information: This information make sure that the tasks and operations are carrying out according to the strategy. This includes that the tasks are completing on time and things are going in a proper manner.
Now, as you have an understanding of the data and information. Let me explain the ten key difference points between both with some real-life examples.
Difference between Data and Information
1. Significance
The very first key difference between the data and information depends on the significance. Information is significant. Whereas, the data is not significant.
It means that the stand-alone data is of no use. There is no meaning that can be derived from raw data and it cannot be utilized anywhere.
On the other hand, the Information is significant as it has some context and provides some meaning. With the information allows taking some action on behalf of it.
With a meaningful data i.e Information, an organization or a business entity can take a decision.
For example, The costs and selling statistics of a product of an E-commerce website when presented in the raw tabular form is not significant. But, when this data is represented within the context of the target customer and the behavior of the customer of purchasing or not purchasing the product. Then this stats become significant as a decision can be taken out on this information.
2. Representation
You can visualize data in a structured form such as tabular data, data tree, data graph etc…
In the tabular format, there is a table with different rows and columns and each column or row represent a data entity.
The data tree format stimulates a hierarchical tree structure with a root node and a number of child nodes.
A data graph is a graphical representation of the data as a bar chart, line chart or a pie chart. The figure below depicts the data graph.
data-representation
Now, coming to the point of information. Information is seen as Language, ideas, and thoughts that are based on the data.
3. Form
The data is in raw form. Basically, the data is in the form of Numbers, letters or a set of characters. It also includes the symbols, picture or audio data. This raw data is scattered and is not aligned with some context.
Whereas the Information is in the form of idea and inference or conclusions that are based on the data. The raw data is analyzed and organized in the whatever context and only the necessary data is kept and the rest is discarded.
For instance, consider the below number:
02011994
This figure is a data entity and doesn’t provide any meaning.
To convert this data into information we need to keep it in some context. Let’s think of a context like a birthdate – 02/01/1994 i.e; Jan 02 1994.
You can interpret the same number as an account number or a mobile number.
4. Reliability
If we talk in the term of reliability then obviously the information wins on this. The information is reliable as it conveys some meaning and there are proper organization and dedication to a single context.
On the other hand, as data is raw and can be provided in any context. Moreover, with every context and structure the output or the meaning of the data changes. Hence, the data is unreliable when compared to Information.
Consider the example above of the number 02011994.
When it is in the form of the date of birth i.e; 02/01/1994 then it depicts a straightforward meaning. This has a clear context.
But if we consider the number only, then it converts into any form and provides a number of meanings that change according to the context.
5. Dependency
In the terms of dependency, the data is independent. As you know the data is raw and data can contain anything. Hence, the data does not depend on any sort of condition or circumstances. It can stand alone.
But, the information depends completely on the data. You cannot process the information witout the data. Data is the very basic building block of the information.
If there is no data then there will be no information.
6. Input and Output
data-and-information-input-process-and-output-5-728
This is the easiest way to differentiate between the two. Data is something that you give as an input for processing. After processing what you get as output is the information.
Let’s take an example of a collage maker application. You provide different photographs that you want to arrange in the collage as the input to the application. After providing the images, the images are processed and aligned according to the chosen theme. In the end, You get a single collage image as the output from the application.
7. Decision Making
You cannot take a decision based on the data while you can take a decision based upon the information.
To take a decision on a situation the very first thing is that you must know and understand the conditions and the circumstances correctly. This is possible only if you have the correct information.
Information plays a vital role in the process of decision making. The actions that a person takes is based on the information that it has.
But if we say about data, as data is raw and is meaningless so it is useless in decision making. You can not take a decision on the basis of raw fact and figures. And if you do so then there is a high priority that the decision may be wrong as it will rely on assumptions.
8. Based on observations
Data is based on the observations and records. The statistics and the tables of collections of figures are the sources of data.
And the source of information is data. Moreover, the information is based on the context that is in the alignment of data.
9. Analysis
The Data is never analyzed in its initial form. Once data is analyzed nor the analysis of data is done, the data becomes information at the same moment.
It means that the information is always analyzed. whereas, the data is never in the analyzed form. Once data is analyzed it is converted into information.
10. Usefulness
The last term of differentiation is the matter of usefulness. Both the data and information are useful in their own terms as the data is the base to create the information. Without the data, there is no information.
But if you see it from the perspective of a business. Then, the data is not so much important as there is a whole lot of processing needs to be done on data to make it useful or understand something out of it.
On the other hand, the Information is always useful as it provides some meaning for further decision making.
Hence, the information is always way more useful than the data.
First Things First: Data vs Information
There’s a really simple way to understand the difference between data and information. When we understand the primary function of the item we are looking at, we quickly see the distinction between the two.
Here’s a simple way to tell one from the other:
- Computers need data. Humans need information.
- Data is a building block. Information gives meaning and context.
In essence, data is raw. It has not been shaped, processed or interpreted. It is a series of 1s and zeros that humans would not be able to read (and nor would they want to). It is disorganised and unfriendly.
Once data has been processed and turned into information, it becomes palatable to human readers. It takes on context and structure. It becomes useful for businesses to make decisions, and it forms the basis of progress.
While the bigger picture is slightly more complex, this gets us part way towards understanding what data means.
The Bigger Picture
When we look at the relationship between data and information, we can establish a larger chain. This is the DIKW Pyramid.
Why DIKW? It stands for Data, Information, Knowledge, Wisdom, and describes the hierarchy between all four.
The DIKW Pyramid describes the acquisition of data, its processing, retention and interpretation, and it’s as applicable to businesses as it is to the human brain.
To see the DIKW Pyramid in action, consider the following example.

- Data: I have one item. The data displays a 1, not a zero.
- Information: It’s a tomato. Now, we understand the item and its characteristics.
- Knowledge: A tomato is a fruit. We can identify patterns in the information and apply them to the item.
- Wisdom: Tomato is never added to a fruit salad. There is an underlying, commonly understood principle that governs the item’s purpose.
Data Quality: The Building Block
In this article, we have truly put data in context. We now understand its position as the foundation. It is the base of a pyramid; the beginning of a continuum.
If data is flawed, the DIKW Pyramid breaks down. The information we derive from the data is not accurate. We cannot make reliable judgments or develop reliable knowledge from the information. And that knowledge simply cannot become wisdom, since cracks will appear as soon as it is tested.
Bad data costs time and effort, gives false impressions, results in poor forecasts and devalues everything else in the continuum.
Data quality software addresses problems with data to avoid these kinds of problems. It ensures that data processing results in reliable information that improves response and retention. This information unlocks the potential of marketing campaigns, increases sales, improves accuracy and adds value.
Data is raw, unanalyzed, unorganised, unrelated, uninterrupted material which is used to derive information, after analyzation. On the other hand, Information is perceivable, interpreted as a message in a particular manner, which provides meaning to data.
Data doesn’t interpret anything as it is a meaningless entity, while information is meaningful and relevant as well. Data and Information are different common terms which we frequently use, although there is a general interchangeability between these terms. So, our primary goal is to clarify the essential difference between Data and Information.
Content: Data Vs Information
- Comparison Chart
- Definition
- Key Differences
- Conclusion
Comparison Chart:
BASIS FOR COMPARISON | DATA | INFORMATION |
Meaning | Data is unrefined facts and figures and utilized as input for the computer system. | Information is the output of processed data. |
Characteristics | Data is a individual unit which contains raw material and doesn't carry any meaning. | Information is the product and group of data which collectively carry a logical meaning. |
Dependence | It doesn't depend on Information. | It relies on Data. |
Peculiarity | Vague | Specific. |
Measuring Unit | Measured in bits and bytes. | Measured in meaningful units like time, quantity, etc. |
Definition of Data :
Data is distinguishable information that is arranged in a particular format. Data word stems from a singular Latin word, Datum; its original meaning is “something given”. We have been using this word since 1600’s, and data turn into the plural of datum.
Data can adopt multiple forms like numbers, letters, set of characters, image, graphic, etc. If we talk about Computers, data is represented in 0’s and 1’s patterns which can be interpreted to represent a value or fact. Measuring units of data are Bit, Nibble, Byte, kB (kilobytes), MB (Megabytes), GB (Gigabytes), TB (Terabytes), PT (Petabyte), EB (Exabyte), ZB (Zettabytes), YT (Yottabytes), etc.
To store data, earlier punched cards were used, which were then replaced by magnetic tapes and hard disks.
There are two variants of data, Qualitative and Quantitative.
- Qualitative Data emerges when the categories present in data are distinctly separated under an observation and expressed through natural language.
- Quantitative Data is the numerical quantification which includes the counts and measurements and can be expressed in terms of numbers.
Data deteriorates as time passes.
Definition of Information :
Information is what you get after processing data. Data and facts can be analysed or used as an effort to gain knowledge and infer on a conclusion. In other words, accurate, systematize, understandable, relevant, and timely data is Information.
Information is an older word that we have been using since 1300’s and have a French and English origin. It is derived from the verb “informare” which means to inform and inform is interpreted as to form and develop an idea.
Information = Data + Meaning
Unlike data, Information is a meaningful value, fact and figure which could derive something useful.
Let us take an example “5000” is data but if we add feet in it i.e. “5000 feet” it becomes information. If we keep on adding elements, it will reach the higher level of intelligence hierarchy as shown in the diagram.
- Information is critical in a sense.
- There are various encoding techniques for interpretation and transmission of information.
- Information encryption is used for increasing the security during transmission and storage also.
Key Differences Between Data and Information
- Data is a single unit which contains raw facts and figures. In contrast, Information is the collection of useful data,which is able to provide knowledge or insight about particular manner.
- Information is derived from the data and hence, data does not rely upon information, but information does.
- Data is used as Input, which needs to be processed and organized in a particular fashion to generate output, i.e. information.
- Data couldn’t specify anything; there is no relation exists between chunks of data while Information is specific and there exists a correlation.
- Data has no real meaning whereas Information carries certain meaning.
Conclusion :
Data and Information, both the terms we use are a part of intelligence hierarchy and differ in the way that Data is not meaningful, but Information which is formed by the processed data is meaningful in context.
Data: The collection of raw facts and figures is called Data/input.
- Data is an input for computer.
- Data is independent.
- When data is lost, it can't be reproduced.
- Data is meaningless and valueless.
Data is Like: { NY, 550, John, marks }
Information: The processed form of data is called information/output.
- Information is an output from computer.
- Information is dependent on data.
- When information is lost, it can reproduced from data.
- Information is meaningful and valuable.